Note: As an Amazon Associate I earn from qualifying purchases.
Stable Video Diffusion just got released: My thoughts (2023)
Introduction
I just tried Stable Video Diffusion from StabilityAI and it’s blowing my mind. It’s still early days, but the promise of turning simple images into elaborate video sequences has me imagining endless possibilities. There’s a learning curve and some hefty hardware requirements, no doubt. Yet, what has me most excited is the potential for creators of all stripes to embrace this new frontier in storytelling and visual communication.
Prices
Check prices of the NVIDIA Deep Learning GPUs on:
The Impact of Stable Video Diffusion on Content Creation
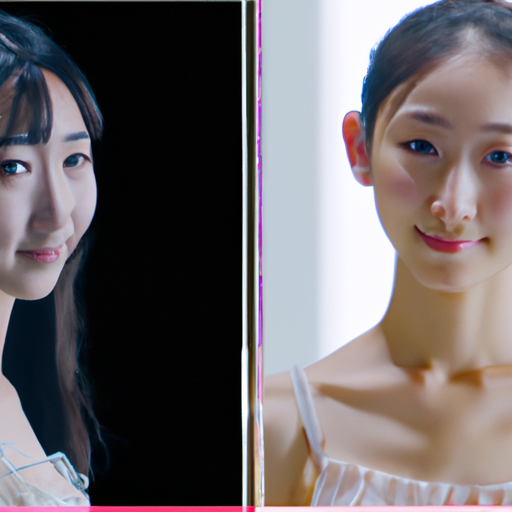
The advent of Stable Video Diffusion (SVD) by StabilityAI represents a significant leap forward in the domain of content creation. As someone who has been closely following the progress of AI diffusion models, the transition from still images to dynamic video generation is a much-anticipated development. The ability to input an image and extrapolate a temporally coherent video sequence opens up new avenues for creators, especially when it comes to storytelling, marketing, and perhaps even prototyping for animation.
From a technical standpoint, SVD is built upon the capabilities of Stable Diffusion, a model that has revolutionized image generation with its stunning output quality and adaptability to various prompts. Details about SVD can be found in the official release paper. The existence of open-source model weights on platforms like Hugging Face hints at the potential for widespread community involvement and rapid iteration that could further refine the technology.
On the practical side, though, we’re still in the early days. Current requirements—like a GPU with a hefty 40 GB VRAM—put SVD out of reach for the average user. It’s a tool that, for the moment, seems destined for well-equipped research labs or professionals with access to high-end hardware. Yet, this feels like a temporary constraint. I’m optimistic that with time, improvements, and the customary ‘slimming down’ process that AI models undergo, we will see more accessible versions. In the meantime, for those who do have access to the necessary equipment, the process can be highly rewarding, as discussed in my experience with Radeon’s RX 7800 XT videocard (2023).
Despite the technical barriers, the potential applications are immense. For individual creators and small studios, SVD could democratize video production, lowering the barriers to creating high-quality visual content. I can envision a future where generating a compelling video could be as straightforward as describing the scene in text form—a future where video editing and animation could be profoundly transformed by artificial intelligence.
There are also potential pitfalls, most notably around the coherence and accuracy of generated content. Current SVD outputs still fall short regarding physical accuracy, spatial consistency, and lighting—a common struggle for AI-generated content that could momentarily limit its applicability to certain genres and styles. Yet, I’m heartened by the rapid progress made in image synthesis and have no doubt these challenges will be tackled in due course.
In summary, while SVD has its limitations, the trajectory of AI-enabled content generation is undeniably upward, with tools getting more powerful and user-friendly. It feels like we’re on the cusp of a new era where, perhaps sooner than we think, creating a video from thin air could be as routine as snapping a photograph today. This is an exciting time to be involved in content creation, indeed.
Technical Challenges and Limitations of Current Models
It’s incredible to see machine learning models now not just interpreting static images, but also dictating motion and narrative in video—that’s a complex dance of pixel prediction over time, which requires substantial computational resources.
What impresses me the most is the sheer speed with which these technologies are iterating. If you told me a few years back we’d soon have models understanding the context of videos, deducing motion from still images and the implications of said motion, I’d have been skeptical. Yet, here we are; the technical prowess on display is hard to dismiss. On the flip side, the hardware requirements to run something like Stable Video Diffusion are nothing short of gargantuan—a GPU with 40GB of VRAM isn’t exactly household standard. This means, for now, the technology remains in the hands of those with access to top-tier equipment or cloud computing resources.
However, it’s imperative to view these hardware requirements as a snapshot in time. If the path of progression in technology has taught us anything, it’s that today’s resource-hungry tech can become tomorrow’s smartphone feature. Efforts like RunPod’s hosting (source) or ComfyUI (source)—a tool that made Stable Diffusion models more accessible—are already underway, ensuring broader access and practical integration.
The major issue I see is related to temporal coherence—the ability of these AI to naturally transition from one frame to the next, maintaining consistency in lighting, structure, and momentum. While the models are getting better, we’re still grappling with oddities such as lighting inconsistencies and structural inaccuracies that any 3D artist or photographer could spot a mile off. The AI videos often come across as slightly off, and it’s these subtle imperfections that will be the hardest to iron out.
Despite the present limitations, the open-source nature of many of these projects, like StabilityAI and Hugging Face, cannot be overstated. The accessibility of these models presents opportunities for community-led innovation, peer review, and rapid improvement that proprietary systems simply cannot match.
I recognize that rollout of features like “move the bicycle to the left side of the photo” is a complex request for current models, but the community is inching closer to these functionalities. Tools like Emu Edit and LLaVa-interactive propose fascinating ways to interact with and iterate upon images with textual prompts, which could extend to video in the not-so-distant future.
In the end, I believe the true potential of AI-generated video lies not just in the heavy lifting of rendering frames, but in the nuanced control and editing options they afford creators. The advancements are definitely a boon, and they give me optimism that with further refinement, we will overcome current technical challenges and usher in a new, more accessible era of video production.
Future Directions and the Evolving Landscape of AI-Generated Media
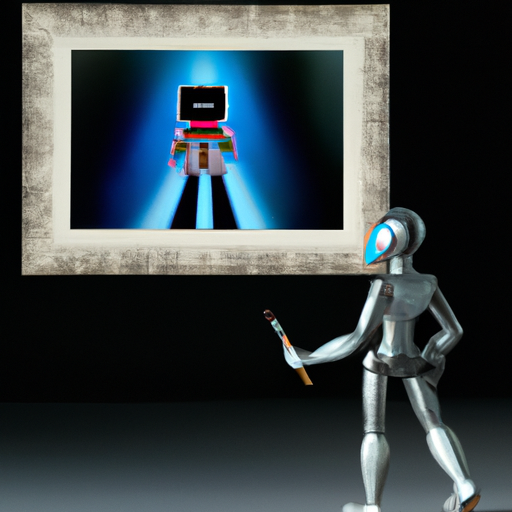
While the requirement of a 40GB GPU initially seems prohibitive—alienating the average user—the tech community is buzzing with optimism. The GitHub discussions around the project reflect a shared excitement and a collective drive to improve accessibility.
I find myself especially intrigued by the potential for iterative models that can manipulate elements within a scene upon user instruction, like moving an object or altering lighting. This would greatly enhance the creative process, making AI a more interactive tool rather than a one-off generator. Meta’s Emu Edit and Emu Video models are pioneering this effort, showing that we’re closer than ever to seamless AI interaction in media creation.
Despite the significant hardware demands and current limitations, there’s promise in the fact that today’s computational luxuries often become tomorrow’s consumer-level norms. It’s not farfetched to anticipate a future where consumer hardware can readily support these models, just as we’ve seen with previous tech trends.
However, we must acknowledge that today’s AI-generated videos still struggle with coherence and physical fidelity—a glaring issue for those within the professional 3D art and film industries. Yet, I can’t shake off the feeling that these are mere speed bumps on the path to an integrated AI-creative suite that hands over control to humans when finesse is needed. The concept of AI as a tool rather than an autonomous creator is a central idea driving many discussions within the community. For example, Blender, a popular open-source 3D software, could greatly benefit from AI that understands and interprets user commands to modify scenes—a powerful avenue that calls for exploration. In the realm of software development, I’ve also shared some insights on incorporating AI into programming workflows as seen in Starting out as a graphics programmer in 2023: My thoughts.
While the models themselves are fascinating, so too are the implications for data. As we’ve seen with Stable Video Diffusion, the need for large datasets seems to be a recurring theme, raising questions about the availability and creation of public datasets. The ObjaverseXL dataset stands out as a notable example of comprehensiveness in the 3D object space, but we are still far from having enough publicly accessible, high-quality 3D scene data to train more robust models. For more insights on the importance of data quality, check out the article on Cleaning Up the Data Mess: The Real Hero in Machine Learning.
Platforms like Hugging Face, a central hub for sharing models and research, are instrumental in fostering open collaboration that pushes the bounds of what generative media can do. The kind of crowd-accelerated innovation we witness there speaks to the power of open-source efforts in the AI landscape. This openness not only democratizes access but accelerates iteration and learning.
In conclusion, while the current landscape is riddled with technological giant leaps that seem just out of reach, it’s the persistent and iterative nature of both AI and its human co-creators that inspires most. The journey to sleeker, more refined, and accessible tools will be one of trial, error, and community. It’s a journey I look forward to—both as an observer and an active participant in this dynamic chapter of creative evolution.